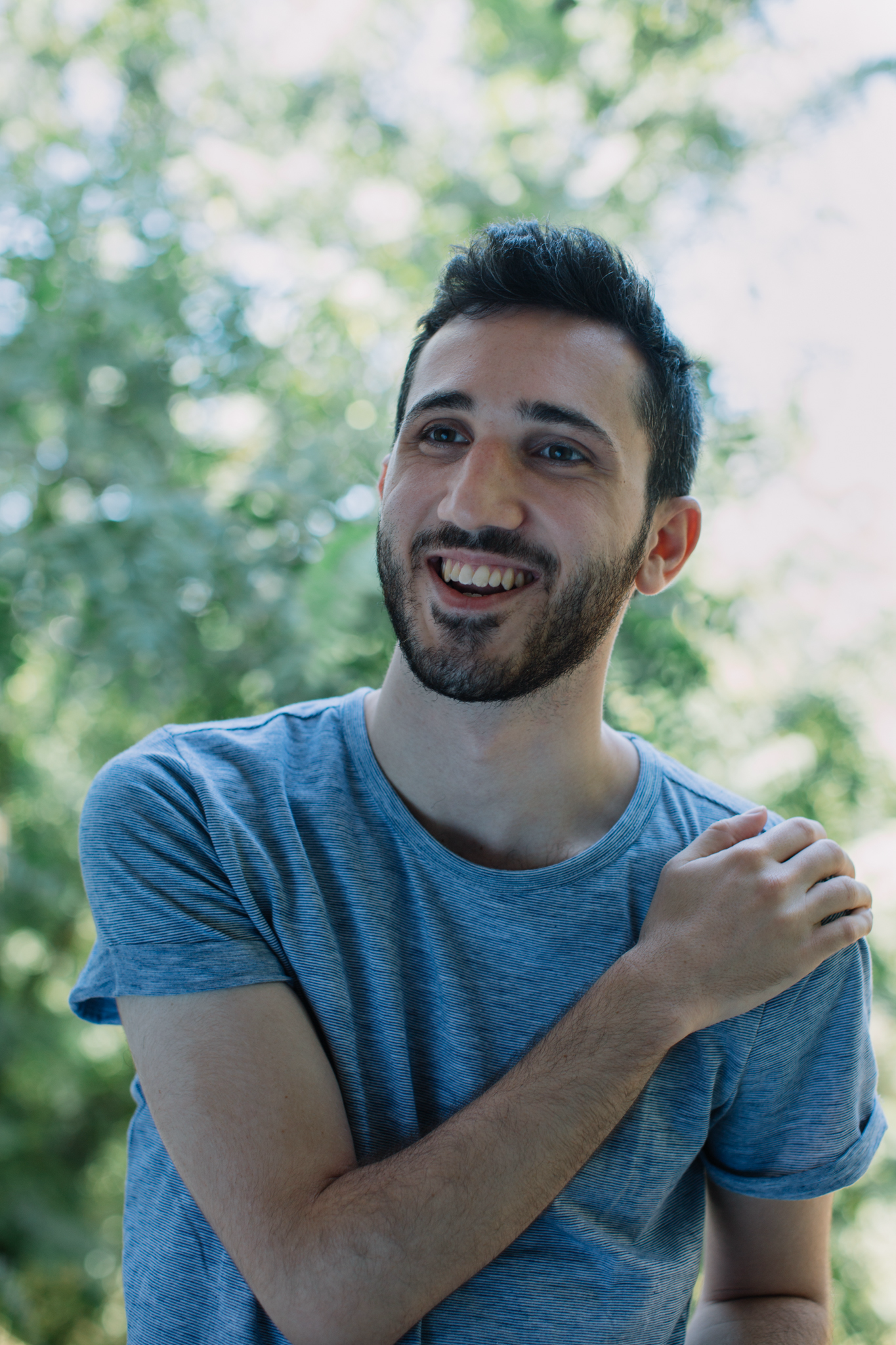
Yuval Ben Dror, Data Science Researcher at Earnix
The applications and potential of AI in insurance are infinite, with opportunities to completely revolutionize an outdated industry full of antiquated processes. Insurers are optimistic about incorporating AI and machine learning (ML), but the evolution has only begun.
One of the key applications of AI for insurers is intelligent monitoring. Continuously monitoring KPIs and concurrently checking for changes in the data and performance of the various models prevents errors by alerting the business of any changes it detects in real time. While this use case is unanimously agreed upon as a positive application of the technology, only a small portion of insurers have implemented it according to an independent survey commissioned by Earnix in 2023.
The two main considerations with automated intelligent monitoring are why it’s so important and conversely, what makes it so difficult to implement. The best way to explain the application of this technology is through an example.
Meet David.
David works in the monitoring team of an insurance company. David is a hard-working individual – once a week, he goes into the company’s monitoring dashboard to monitor the KPIs defined by his managers to detect any significant drifts in performance. To do this, he investigates the issue by performing extensive research and data segmentation. However, by the time he feels confident in the main causes for the drifts he observes, the performance sometimes reverts to its original trend. In other cases, the KPIs have already suffered a significant hit, which could have been addressed much earlier if detected in time.
Why does it take him so long? Multiple factors contribute to the process being exhaustive and time-consuming. First, he must identify the drift, which is far from trivial. He must find a point of comparison and clearly understand the targets before deciding whether they are being met. Then, to identify the cause of the change, he must perform elaborate research in data tables that are usually large and could contain hundreds, sometimes thousands, of column transformations that go into predictive models.
Current monitoring solutions typically offer dashboards that allow you to perform a vast number of multivariate segmentations. What they don’t offer is a clear expected trend or expected data distribution that can be used as a point of comparison, and a precise, to-the-point dashboard that guides you towards the main drivers behind the detected changes. Therefore, they still require a lot of manual work and can’t really be considered automatic.
Automated intelligent monitoring could significantly shorten David’s work by identifying the drifts and performing an initial analysis to flag the potential causes. That data analysis, especially related to causal inference, is prone to error, particularly when done automatically. However, when applied correctly, there are tools that could help point the analyst in the right direction and significantly reduce the time needed to perform the analysis.
Intelligent monitoring is just one example of how advanced automation technologies are furthering what insurers can do. But, by implementing these kinds of changes, companies can be much more reactive to market changes, able to adapt quicker to the changing reality, and ensure they are doing everything in its power to hit the targets they set.
Yuval Ben Dror, is the Data Science Researcher at Earnix